爱来自ZZULI❤drluo
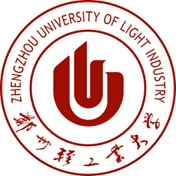
1. Config 类定义¶
Config
类为用于配置构建 Predictor
对象的配置信息,如模型路径、是否开启 gpu 等等。
构造函数定义如下:
1 2 3 4 5 6 7 8
| # Config 类定义,输入为 None class paddle.inference.Config()
# Config 类定义,输入为其他 Config 对象 class paddle.inference.Config(config: Config)
# Config 类定义,输入分别为模型文件路径和参数文件路径 class paddle.inference.Config(prog_file: str, params_file: str)
|
代码示例:
1 2 3 4 5 6 7 8
| # 引用 paddle inference 预测库 import paddle.inference as paddle_infer
# 创建 config config = paddle_infer.Config("./mobilenet.pdmodel", "./mobilenet.pdiparams")
# 根据 config 创建 predictor predictor = paddle_infer.create_predictor(config)
|
2. 设置预测模型¶
2.1. 从文件中加载预测模型¶
API定义如下:
1 2 3 4 5 6 7 8 9 10 11 12 13 14 15 16 17 18 19 20 21 22 23 24 25
| # 设置模型文件路径,当需要从磁盘加载模型时使用 # 参数:prog_file_path - 模型文件路径 # params_file_path - 参数文件路径 # 返回:None paddle.inference.Config.set_model(prog_file_path: str, params_file_path: str)
# 设置模型文件路径 # 参数:x - 模型文件路径 # 返回:None paddle.inference.Config.set_prog_file(x: str)
# 设置参数文件路径 # 参数:x - 参数文件路径 # 返回:None paddle.inference.Config.set_params_file(x: str)
# 获取模型文件路径 # 参数:None # 返回:str - 模型文件路径 paddle.inference.Config.prog_file()
# 获取参数文件路径 # 参数:None # 返回:str - 参数文件路径 paddle.inference.Config.params_file()
|
代码示例:
1 2 3 4 5 6 7 8 9 10 11 12 13 14 15 16
| # 引用 paddle inference 预测库 import paddle.inference as paddle_infer
# 创建 config config = paddle_infer.Config()
# 通过 API 设置模型文件夹路径 config.set_prog_file("./mobilenet_v2.pdmodel") config.set_params_file("./mobilenet_v2.pdiparams")
# 通过 API 获取 config 中的模型文件和参数文件路径 print(config.prog_file()) print(config.params_file())
# 根据 config 创建 predictor predictor = paddle_infer.create_predictor(config)
|
2.2. 从内存中加载预测模型¶
API定义如下:
1 2 3 4 5 6 7 8 9 10 11 12 13
| # 从内存加载模型 # 参数:prog_buffer - 内存中模型结构数据 # prog_buffer_size - 内存中模型结构数据的大小 # params_buffer - 内存中模型参数数据 # params_buffer_size - 内存中模型参数数据的大小 # 返回:None paddle.inference.Config.set_model_buffer(prog_buffer: str, prog_buffer_size: int, params_buffer: str, params_buffer_size: int)
# 判断是否从内存中加载模型 # 参数:None # 返回:bool - 是否从内存中加载模型 paddle.inference.Config.model_from_memory()
|
代码示例:
1 2 3 4 5 6 7 8 9 10 11 12 13 14 15 16 17 18 19 20 21
| # 引用 paddle inference 预测库 import paddle.inference as paddle_infer
# 创建 config config = paddle_infer.Config()
# 加载模型文件到内存 with open('./mobilenet_v2.pdmodel', 'rb') as prog_file: prog_data=prog_file.read() with open('./mobilenet_v2.pdiparams', 'rb') as params_file: params_data=params_file.read()
# 从内存中加载模型 config.set_model_buffer(prog_data, len(prog_data), params_data, len(params_data))
# 通过 API 获取 config 中 model_from_memory 的值 - True print(config.model_from_memory())
# 根据 config 创建 predictor predictor = paddle_infer.create_predictor(config)
|
重要内容
paddlepaddle中
- DynamicDimension :表示动态维度,一般是1
- im_shape:图像经过resize后的大小,表示为H,W, DynamicDimension 表示batch维度
- image:输入网络的图像,DynamicDimension 表示batch维度,如果输入图像大小为变长,则H,W为None
- scale_factor:输入图像大小比真实图像大小,表示为scale_y, scale_x
- multiclass_nms3_0.tmp_0:bbox, NMS的输出,形状为[N, 6], 其中N为预测框的个数,6为[class_id, score, x1, y1, x2, y2]
- multiclass_nms3_0.tmp_2:bbox_num, 每张图片对应预测框的个数,例如batch_size为2,输出为[N1, N2], 表示第一张图包含N1个预测框,第二张图包含N2个预测框,并且预测框的总个数和NMS输出的第一维N相同